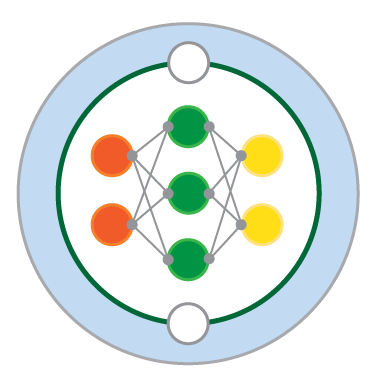 |
Balwada, D., …, Loose, N., and Zanna, L. (2024). Learning Machine Learning with Lorenz-96, Journal of Open Source Education. doi: 10.21105/jose.00241. |
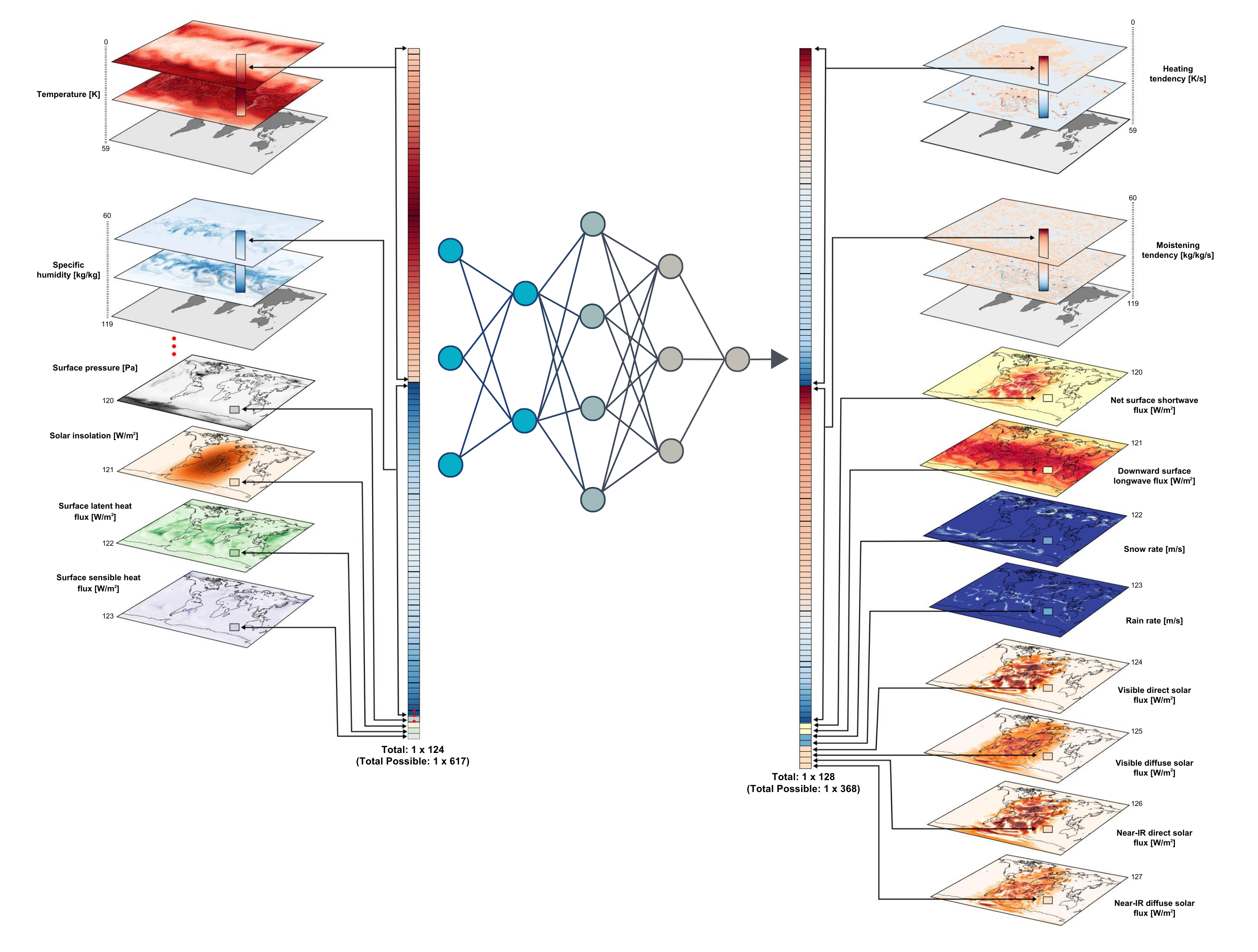 |
Yu, S., …, Loose, N., … and Pritchard, M.S. (2023). ClimSim: A Large Multi-Scale Dataset for Hybrid Physics-ML Climate Emulation. Advances in Neural Information Processing Systems, url. |
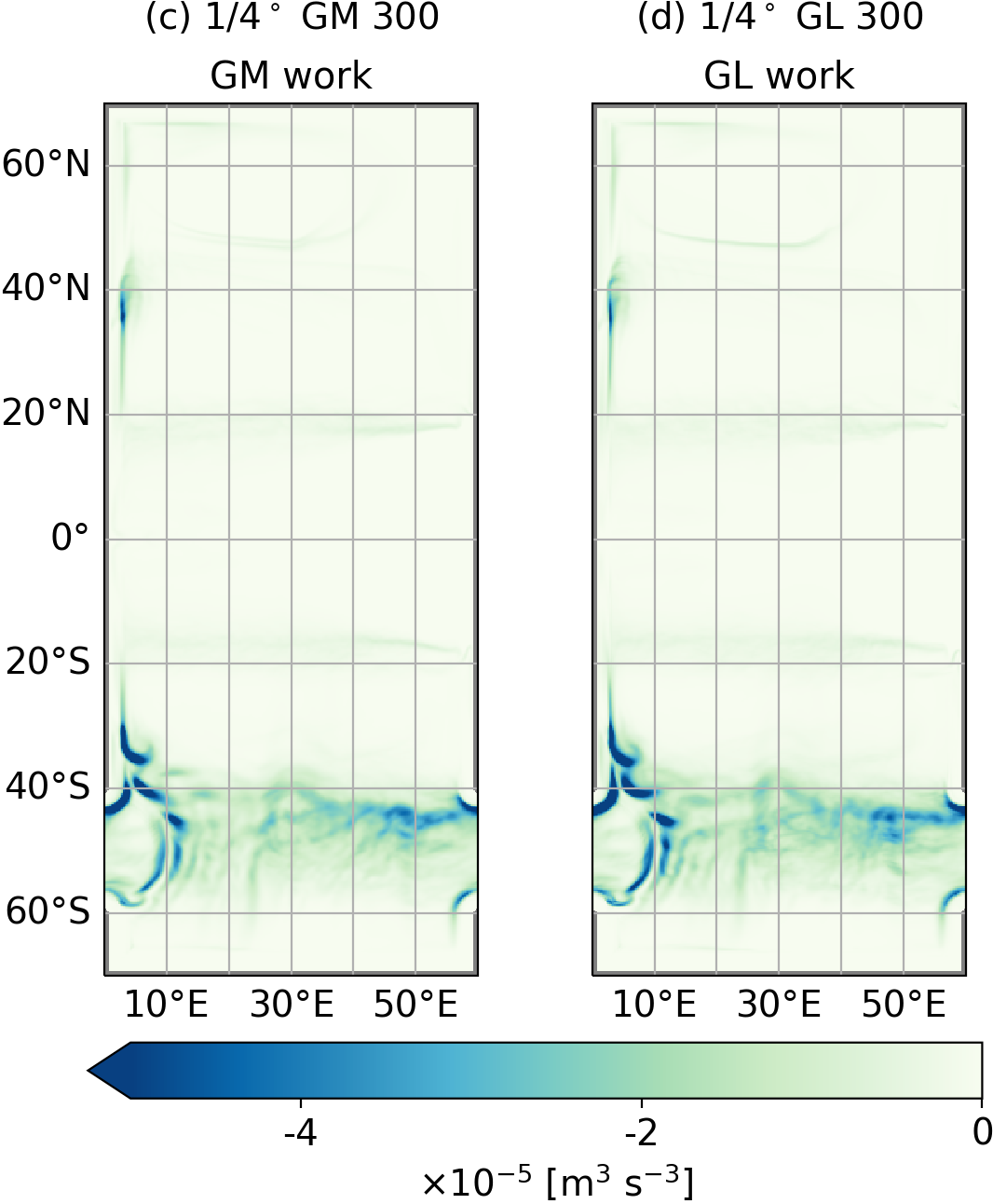 |
Loose, N., Marques, G.M., Adcroft, A., Bachman, S., Griffies, S.M., Grooms, I., Hallberg, R.W. and Jansen, M. (2022). Comparing two parameterizations for the restratification effect of mesoscale eddies in an isopycnal ocean model. Journal of Advances in Modeling Earth Systems. doi: 10.1029/2022MS003518. |
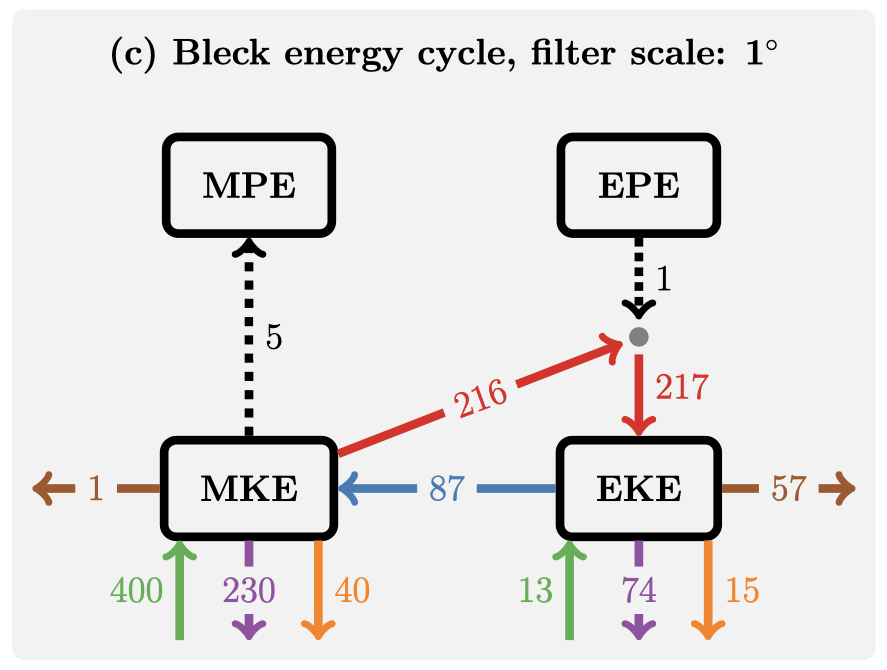 |
Loose, N., Bachman, S., Grooms, I. and Jansen, M. (2022). Diagnosing scale-dependent energy cycles in a high-resolution isopycnal ocean model. Journal of Physical Oceanography. doi: 10.1175/JPO-D-22-0083.1, Open-access preprint: 10.1002/essoar.10511055.2. |
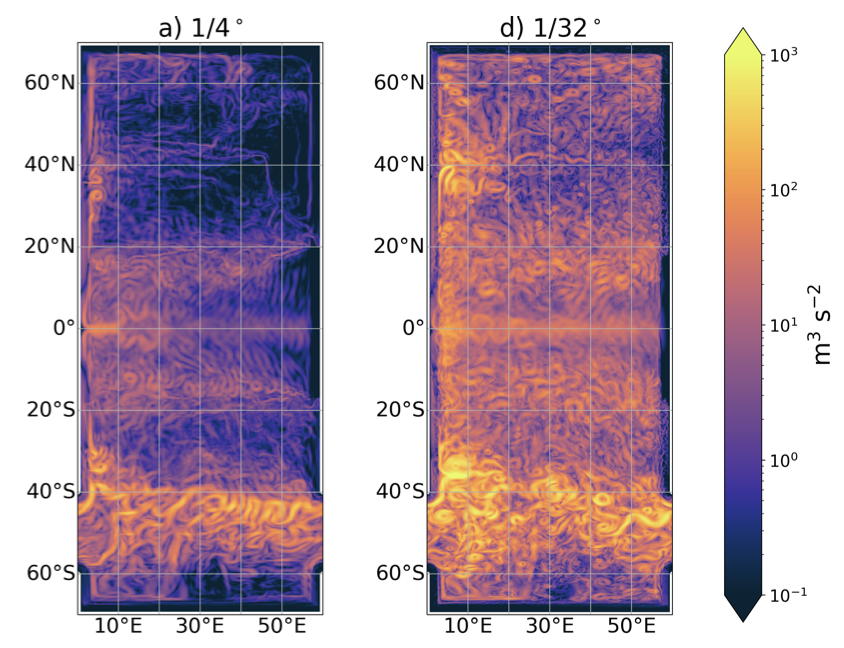 |
Marques, G., Loose, N., Yankovsky, E., Steinberg, J., Chang, C-Y., Bhamidipati, N., Adcroft, A., Fox-Kemper, B., Griffies, S., Hallberg, R., Jansen, M., Khatri, H. and Zanna, L. (2022). NeverWorld2: An idealized model hierarchy to investigate ocean mesoscale eddies across resolutions. Geoscientic Model Development 15, no. 17: 6567-79. doi: 10.5194/gmd-15-6567-2022. |
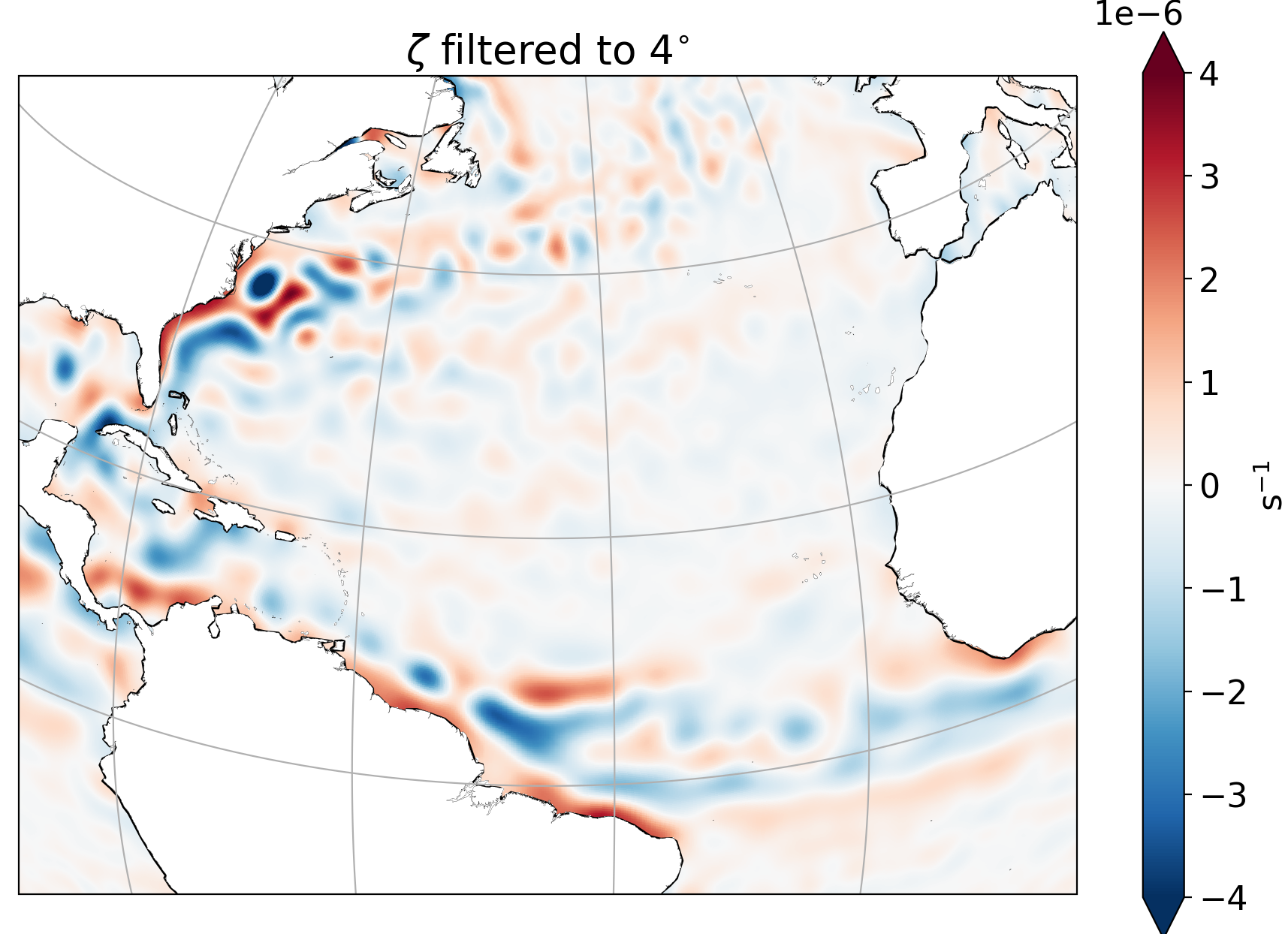 |
Loose, N., Abernathey, R., Grooms, I., Busecke, J., Guillaumin, A.P., Yankovsky, E., Marques, G., Steinberg, J.M., Ross, A.S., Khatri, H., Bachman, S.D., Zanna, L., Martin, P. (2022). GCM-Filters: A Python Package for Diffusion-based Spatial Filtering of Gridded Data, Journal of Open Source Software. doi: 10.21105/joss.03947. |
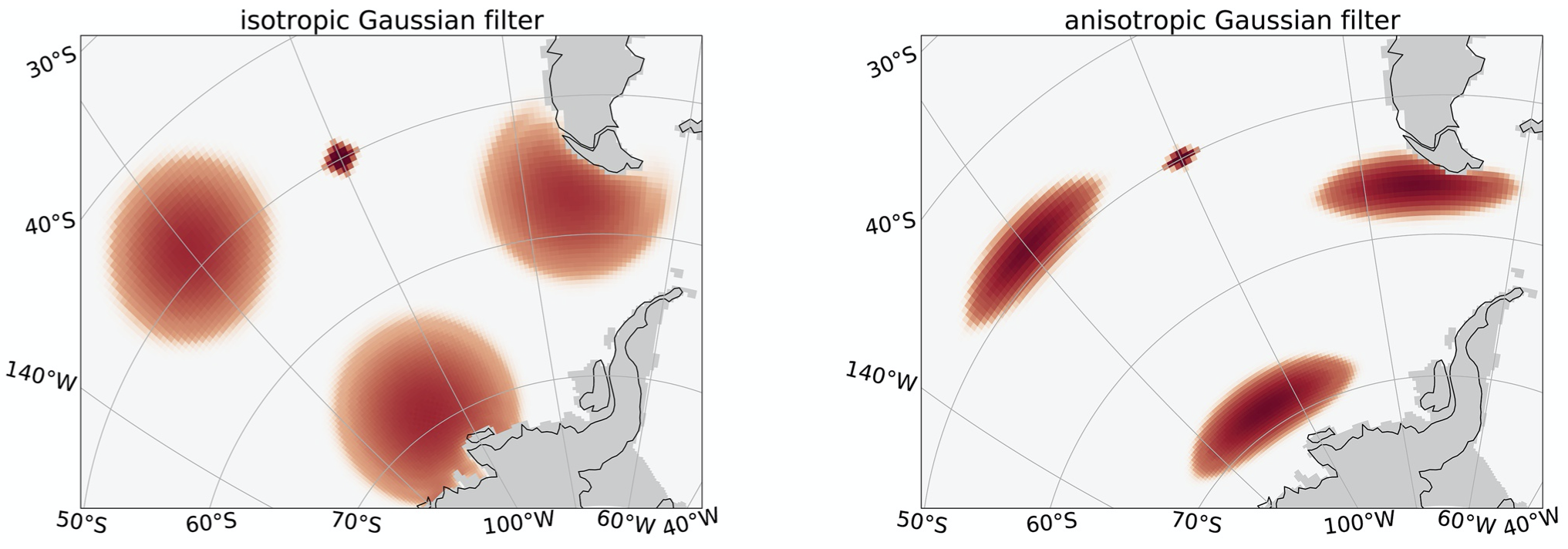 |
Grooms, I., Loose, N., Abernathey, R., Steinberg, J.M., Bachman, S.D., Marques, G., Guillaumin A.P., Yankovsky E. (2021). Diffusion-Based Smoothers for Spatial Filtering of Gridded Geophysical Data, Journal of Advances in Modeling Earth Systems. doi: 10.1029/2021MS002552. |
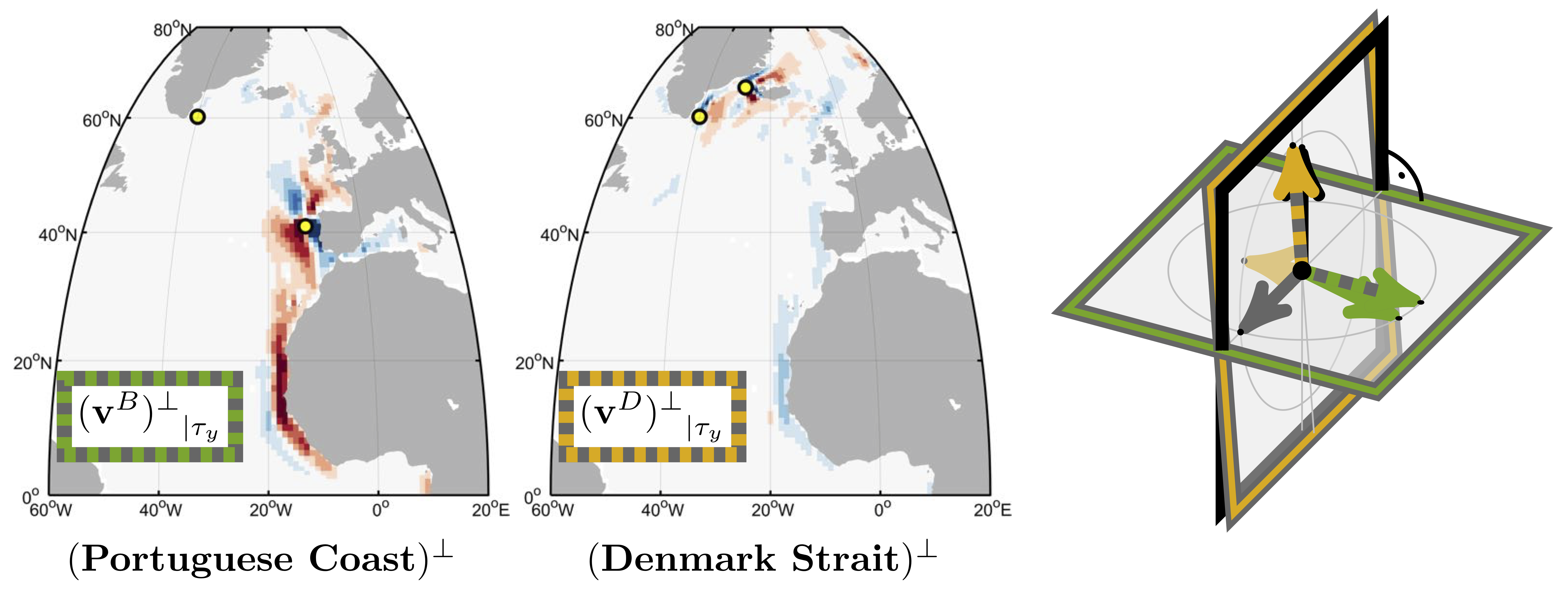 |
Loose, N. and Heimbach, P (2021). Leveraging Uncertainty Quantification to Design Ocean Climate Observing Systems. Journal of Advances in Modeling Earth Systems. doi: 10.1029/2020MS002386. |
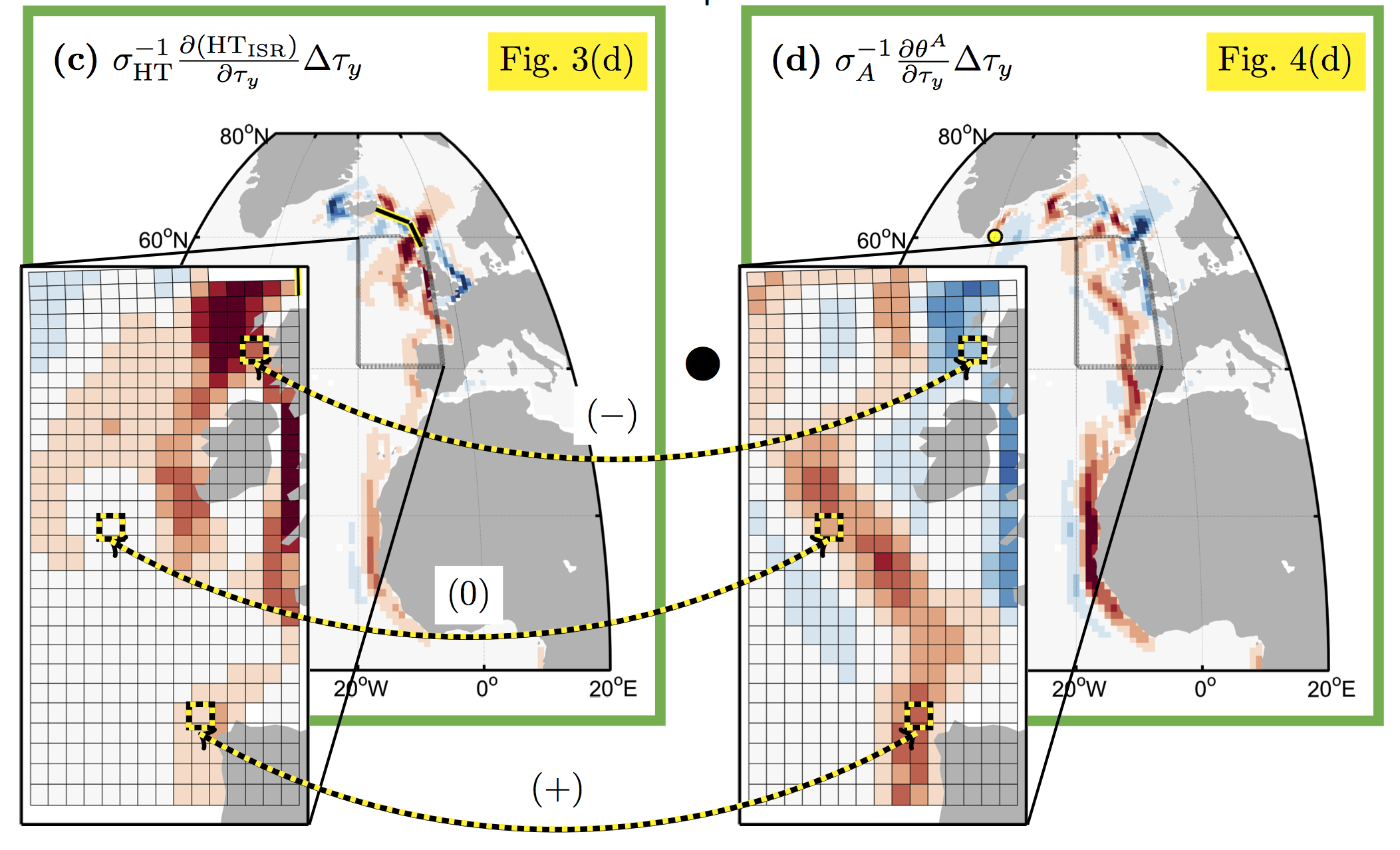 |
Loose, N., Heimbach, P., Pillar, H. and Nisancioglu, K. (2020) Quantifying Dynamical Proxy Potential through Shared Adjustment Physics in the North Atlantic. Journal of Geophysical Research: Oceans 125, no. 9. doi: 10.1029/2020JC016112. Selected as Eos Research Spotlight and highlighted in this StoryMap. |
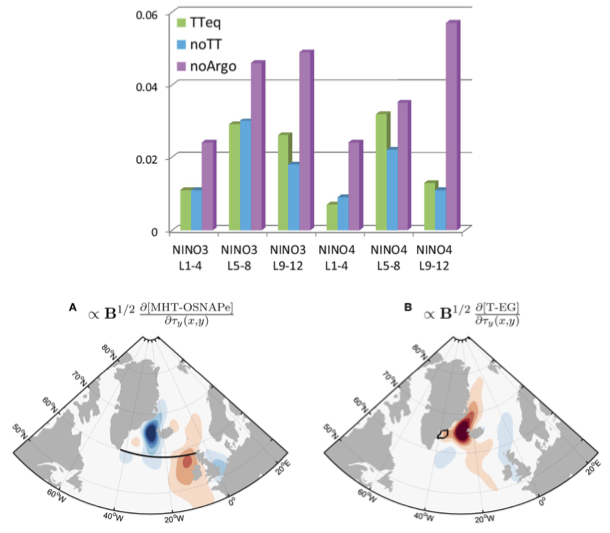 |
Fujii, Y., Remy, E., Zuo, H., Oke, P., Halliwell, G., Gasparin, F., Benkiran, M., Loose, N., Cummings, J., Xie, J., Xue, Y., Masuda, S., Smith, G.C., Balmaseda, M., Germineaud, C., Lea, D.J., Larnicol, G., Bertino, L., Bonaduce, A., Brasseur, P., Donlon, C., Heimbach, P., Kim, Y., Kourafalou, V., Le Traon, P-Y., Martin, M., Paturi, S., Tranchant, B. and Usui, N. (2019). Observing System Evaluation Based on Ocean Data Assimilation and Prediction Systems: On-Going Challenges and a Future Vision for Designing and Supporting Ocean Observational Networks. Front. Mar. Sci. 6:417. doi: 10.3389/fmars.2019.00417 |